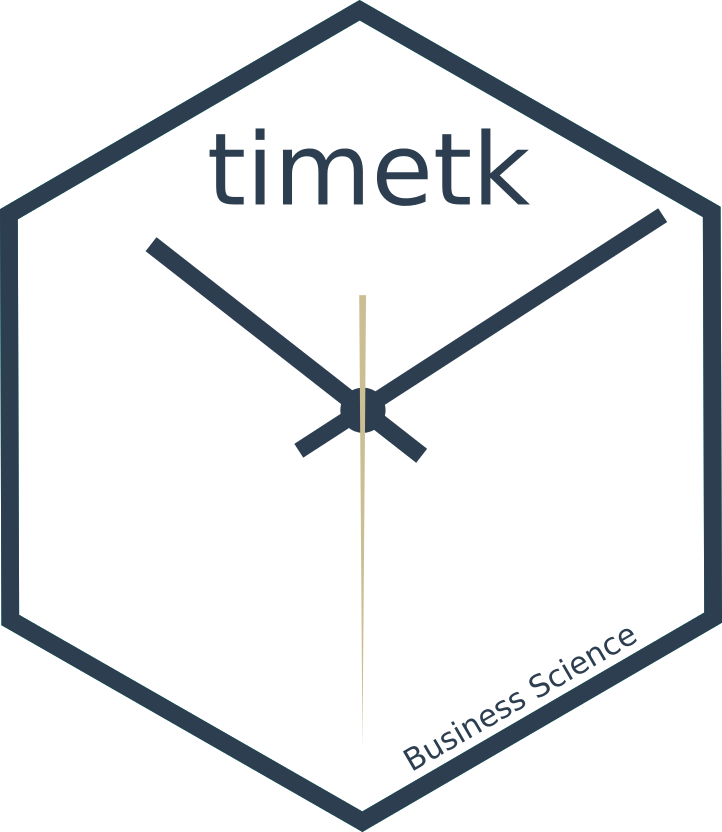
Visualize STL Decomposition Features for One or More Time Series
Source:R/plot-stl_diagnostics.R
plot_stl_diagnostics.Rd
An interactive and scalable function for visualizing time series STL Decomposition.
Plots are available in interactive plotly
(default) and static ggplot2
format.
Usage
plot_stl_diagnostics(
.data,
.date_var,
.value,
.facet_vars = NULL,
.feature_set = c("observed", "season", "trend", "remainder", "seasadj"),
.frequency = "auto",
.trend = "auto",
.message = TRUE,
.facet_scales = "free",
.line_color = "#2c3e50",
.line_size = 0.5,
.line_type = 1,
.line_alpha = 1,
.title = "STL Diagnostics",
.x_lab = "",
.y_lab = "",
.interactive = TRUE
)
Arguments
- .data
A
tibble
ordata.frame
with a time-based column- .date_var
A column containing either date or date-time values
- .value
A column containing numeric values
- .facet_vars
One or more grouping columns that broken out into
ggplot2
facets. These can be selected usingtidyselect()
helpers (e.gcontains()
).- .feature_set
The STL decompositions to visualize. Select one or more of "observed", "season", "trend", "remainder", "seasadj".
- .frequency
Controls the seasonal adjustment (removal of seasonality). Input can be either "auto", a time-based definition (e.g. "2 weeks"), or a numeric number of observations per frequency (e.g. 10). Refer to
tk_get_frequency()
.- .trend
Controls the trend component. For STL, trend controls the sensitivity of the lowess smoother, which is used to remove the remainder.
- .message
A boolean. If
TRUE
, will output information related to automatic frequency and trend selection (if applicable).- .facet_scales
Control facet x & y-axis ranges. Options include "fixed", "free", "free_y", "free_x"
- .line_color
Line color.
- .line_size
Line size.
- .line_type
Line type.
- .line_alpha
Line alpha (opacity). Range: (0, 1).
- .title
Plot title.
- .x_lab
Plot x-axis label
- .y_lab
Plot y-axis label
- .interactive
If TRUE, returns a
plotly
interactive plot. If FALSE, returns a staticggplot2
plot.
Details
The plot_stl_diagnostics()
function generates a Seasonal-Trend-Loess decomposition.
The function is "tidy" in the sense that it works
on data frames and is designed to work with dplyr
groups.
STL method:
The STL method implements time series decomposition using
the underlying stats::stl()
. The decomposition separates the
"season" and "trend" components from
the "observed" values leaving the "remainder".
Frequency & Trend Selection
The user can control two parameters: .frequency
and .trend
.
The
.frequency
parameter adjusts the "season" component that is removed from the "observed" values.The
.trend
parameter adjusts the trend window (t.window
parameter fromstl()
) that is used.
The user may supply both .frequency
and .trend
as time-based durations (e.g. "6 weeks") or numeric values
(e.g. 180) or "auto", which automatically selects the frequency and/or trend
based on the scale of the time series.
Examples
library(dplyr)
# ---- SINGLE TIME SERIES DECOMPOSITION ----
m4_hourly %>%
filter(id == "H10") %>%
plot_stl_diagnostics(
date, value,
# Set features to return, desired frequency and trend
.feature_set = c("observed", "season", "trend", "remainder"),
.frequency = "24 hours",
.trend = "1 week",
.interactive = FALSE)
#> frequency = 24 observations per 24 hours
#> trend = 168 observations per 1 week
# ---- GROUPS ----
m4_hourly %>%
group_by(id) %>%
plot_stl_diagnostics(
date, value,
.feature_set = c("observed", "season", "trend"),
.interactive = FALSE)
#> frequency = 24 observations per 1 day
#> trend = 336 observations per 14 days
#> frequency = 24 observations per 1 day
#> trend = 336 observations per 14 days
#> frequency = 24 observations per 1 day
#> trend = 336 observations per 14 days
#> frequency = 24 observations per 1 day
#> trend = 336 observations per 14 days